Netico energy predictor is a software module built on top of the Hive system and Netico energy monitoring platform, used to predict the future energy consumption of the monitored process. Accurate energy prediction is important in many cases, such as:
• Planning of grid development,
• Optimization and planning of energy trading,
• Monitoring and optimization of renewable energy sources.
Netico energy predictor uses advanced machine learning techniques based on the deep learning recurrent neural network architecture, which allows for flexibility in using different parameters to learn the patterns of energy use.In the basic configuration, Netico energy predictor uses historical consumption records, time and information about holidays and special days to learn the consumption patterns. This can be extended with additional parameters like weather forecast and various process parameters to reduce the prediction error.
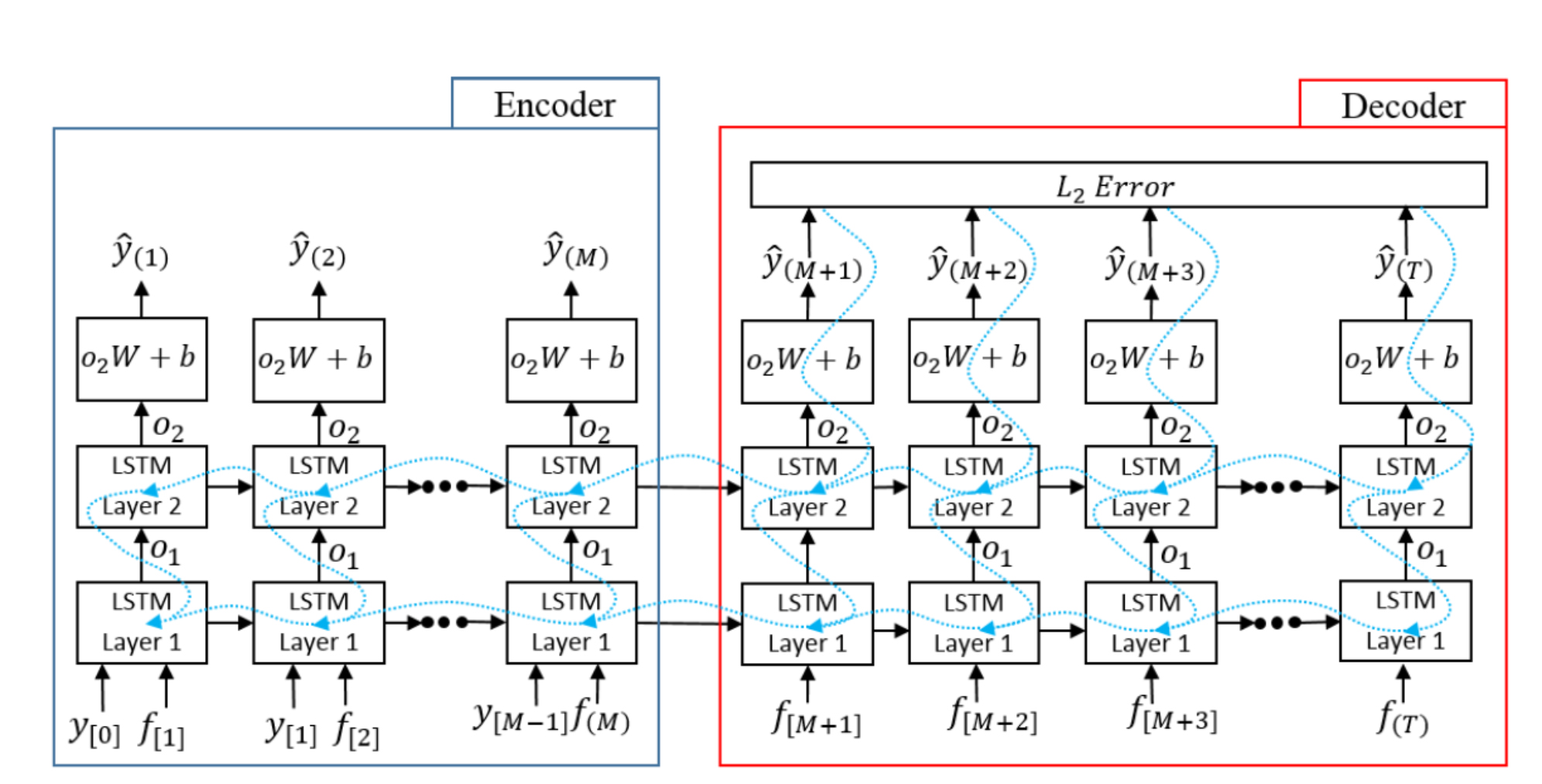
How it works?
Netico energy predictor uses historical energy consumption data collected using Netico NTPM energy sensor or third party devices and combines this information with other parameters that could influence the consumption. Once the basic learning is done, the prediction model is deployed in operation and acts as a virtual measurement equipment, which “measures” and outputs the future energy consumption for a specified time window. Based on this future consumption data, it is possible to configure Netico Hive platform to issue alarms, control equipment or simply just display the information in convenient dashboards for efficient decision making.
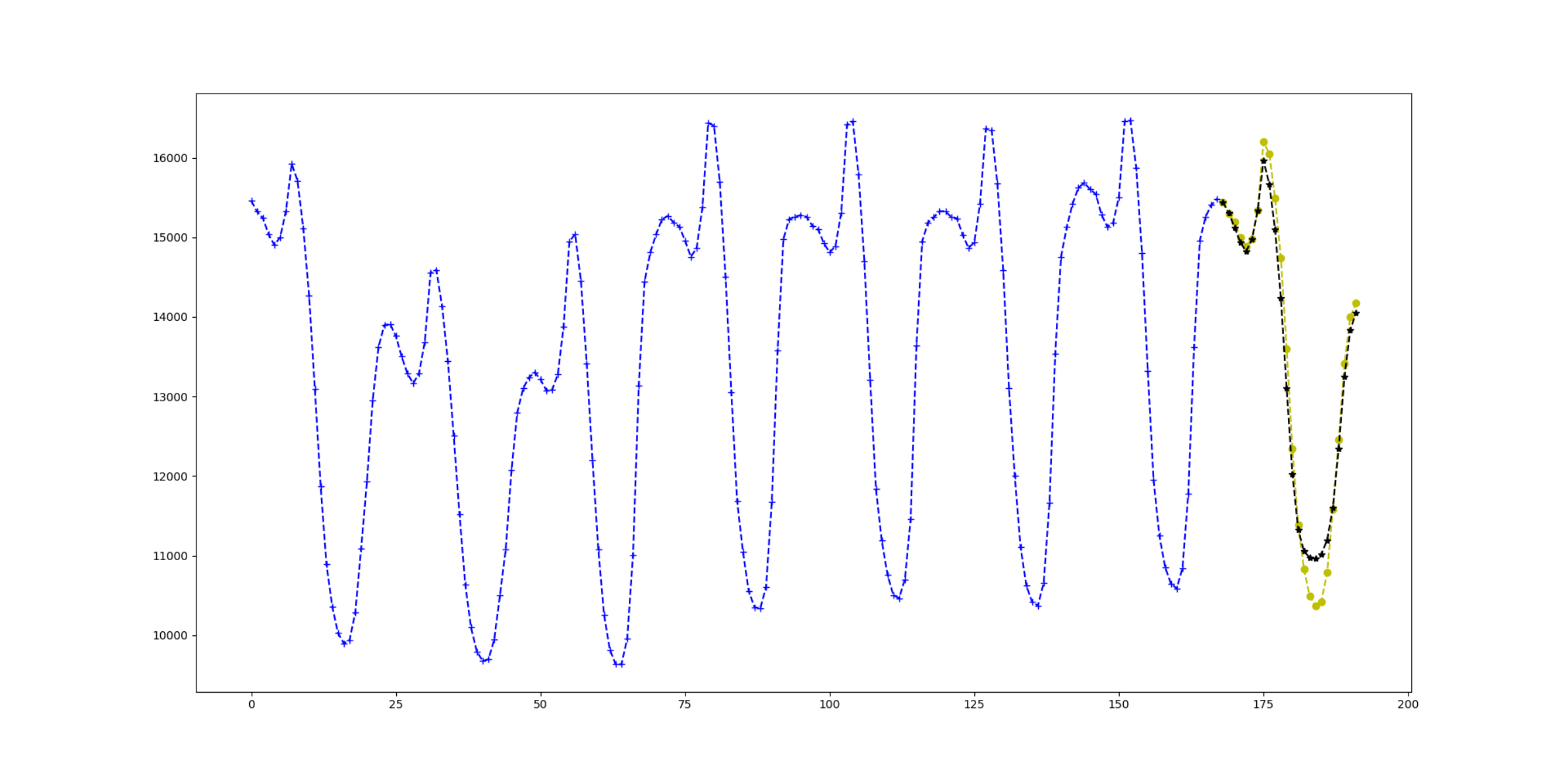
Typical Use cases
• Management of renewable energy sources
• Energy trading optimization
• Optimization of energy supply chain
• Grid planning and development
Deployment
Netico Energy predictor is offered as a service on top of Netico or third party energy management systems. The machine learning training environment is hosted in the Netico cloud, where the training of the prediction model for a specific use case is done. The trained model is then deployed and used as either on-premise or as the cloud-based service, depending on the concrete customer needs. The learned prediction model is constantly updated as the new data is collected to reflect changes in the consumption patterns. Please contact us to discuss the deployment in your specific scenario and for the information about available pricing models.